마케터를 위한 lookalike model
lookalike 모델링이란?
Look-alike modeling is a process that identifies people who look and act just like your target audience.
Look-alike modeling uses machine learning to find more users who will take that action.
This means your campaigns can scale to reach more people with a higher engagement rate.
Lookalike 모델링을 왜 사용해야 할까?
- to seek audiences with behaviors that match up with your target audience
ㄴ greater chance to convert them
How to identify?
The demographic and behavioral data points of anyone who completes a sale and makes it to that purchase confirmation page
시작하기 위해 필요한것?
seed data : goal 을 이미 달성한 그룹
Machine learning will process it and start to look for new potential customers who share some characteristics with them.
look-alike modeling
expanding the size of ad segments -> increased reach
seed set : Which set of users should be the Look alike audience be based off of?
Segment Size: How large should the audience be?
use as set of 1,000 known homeowners (the seed set)
to build an audience of 50,000 Lookalike who are similar to those homeowners.
ㄴ expand the target audience
lookalike models are learned using informaiton about users in the seed set (positive set)
withouht any consideration for users who do not belong in the seed set (negative labels)
Specifically, a lookalike model will search for any trait shared by users in the seed set,
even if that trait is not unique to that set.
seed data 를 고객풀중 registered 한 고객으로 가져가면, 이들이 타겟 고객과 다르게 행동할 수 있단 가능성을 배제하지 못함
ex >
target audience - samll buseinss owners
1M 유저 중
100K 프로필 등록, job title 도 등록
100 K 중에 2K 만 small buseinss owners 임
ㄴ 나머지 8K 에서 small busienss owners 와 유사특징을 가진 사람을 발라냄
Point : seed data 는 registered 풀이기 때문에 이들이 unregistered 고객과 다르게 행동 할 수 있음
오류 :
registered 고객 정보에서 traits 발굴 비슷한 traits 가진 사람들 unresigered 에서 찾음
ㄴ 성과는 저조할 것
lookalike alternatives #1: Classification
위에 케이스로 돌아가서
seed set contained a hidden trait in the form of registered users
ㄴ dominant factor 을 찾아보니 small business owners 과 무관
검증하는 과정 classification
positive set: registered 풀 - small business owners
negative set: registered 풀 - non small business onwers
seed data : 범위를 축소할 수 있음
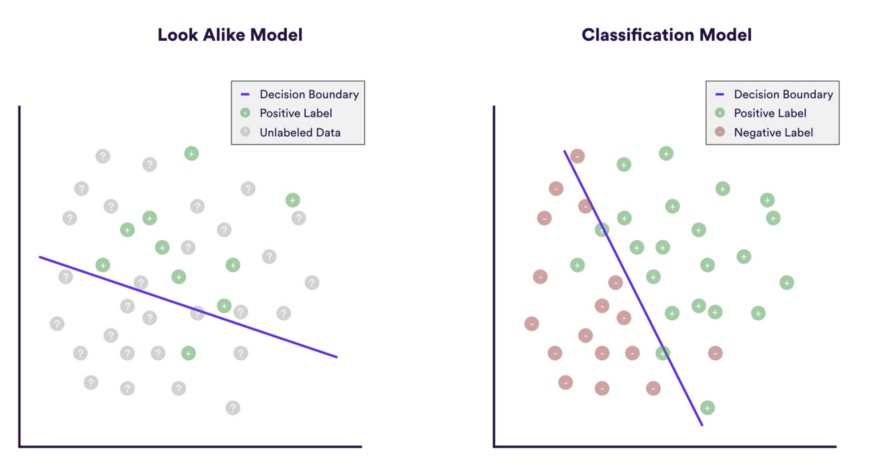
lookalike alternatives #2: Conversion Predictions
위에 케이스로 돌아가서, 은행 대출상품 판매 - target audience 정의 : small business owners 라고 할 때
ㄴ small businessowners : 광고에 관심을 보일 수 있음 but 전환률이나 특정 행동까지 이어지지 않음
(대출에 관심 있거나, 사업을 준비하고 있거나 등)

lookalike alternatives #3: uplift predictions
*uplift - to influence downstream customers - igmv - iroas
Uplift predictions - predict how each customer is likely to respond to a particular intervention
cohort
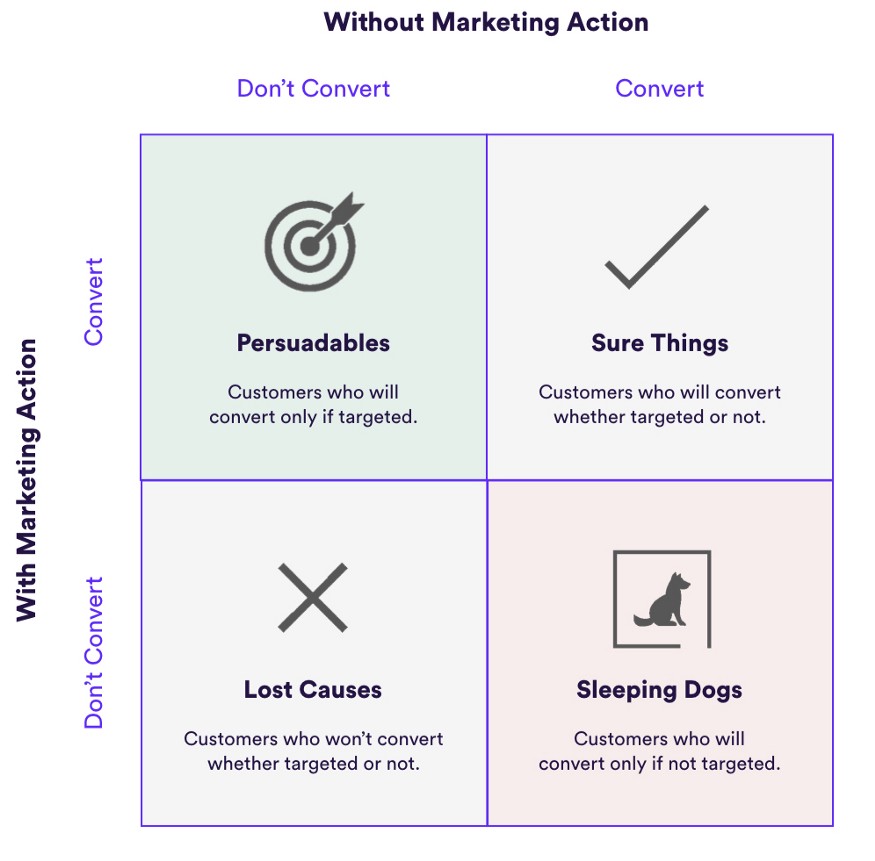
With/without marketing action 으로 don't convert/convert 예측
persuadable/sure things / lost causes /sleeping dogs 네 그룹으로 나눠 타겟함
beauty - vegan
ㄴ 1. lookalike , seed data : vegan 구매 기록 있는 고객
ㄴ 2. vegan 구매 lookalike distance ~0.5
ㄴ output: purchase rate 올라감
look-alike modeling
an effective targeting system that reduces your investments required to acquire new customers and helps your business to expand with a stable business model.
Case study
bank to target prospects for investing with the bank
Having similar traits and behavior like your already existing customers.
" finding lookalike audiences of your best, most profitable customers "
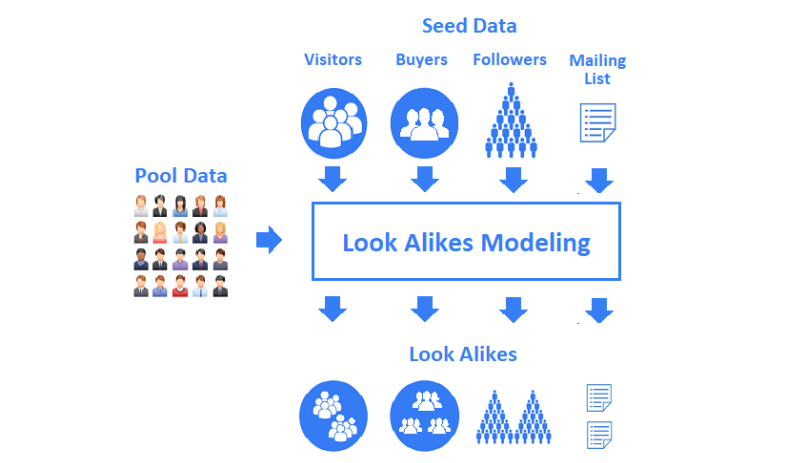
Seed data: 기준 풀
Pool data: 적용풀
extended look-alike audience: 적용해서 추출한 그룹
Benefits
lower aquisition cost -> reduace CAC - only spend marketing efforts on potential customers
seed data : fresh active customers
pool data : fresh eligible but not active customers
extended look-alike audience :
testing: classfication
postive : pool data with traits
neative : pool data without traits
loyal and profitable customer base : high customer lifetime value
seed data : habituated customers
pool data : active but not habituated
extended look-alike :
Case
seed data: 기존 캠페인 데이터 고객
pool data :
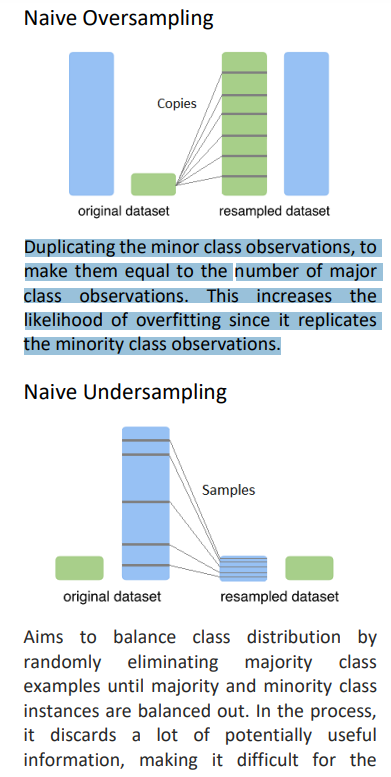
link : https://www.lotame.com/back-basics-look-alike-modeling/
Back to Basics: What is Look-alike Modeling? | Lotame
Look-alike modeling is something every marketer should be an expert on, especially if their goal is to reach greater audiences. What is look-alike modeling?
www.lotame.com
Data Science for Ad Segments : Moving Beyond Look-Alike Modeling
Segment Classification, Conversion Predictions, and Uplift Modeling
towardsdatascience.com
link: https://www.perceptive-analytics.com/wp-content/uploads/2019/11/LookAlikes_Case_Study_Ver1.0.pdf
Objective
makes targetting more effective and precise so, which reduces CAC
'마케팅' 카테고리의 다른 글
이탈고객 재획득 (0) | 2023.02.27 |
---|---|
온라인 MBA 지원방법과 절차 그리고 혜택 (0) | 2023.02.27 |
strategy for win back lapsed customers (0) | 2023.02.26 |
세그먼트 전략 | 마케터라면 알면 좋은 recommendation modeling (0) | 2023.02.17 |
CRM 마케터 다이어리 | 2023년 주요 마케팅 트렌드 키워드 정리 (0) | 2022.12.10 |